The Problem of Cumulative Advantage
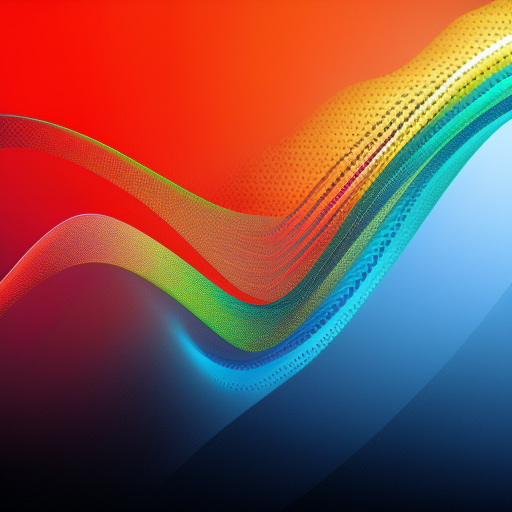
"Cumulative advantage" is when small initial successes compound over time to create bigger later advantages. But, whether a particular ad gets a success early, or late, is partially random. So cumulative advantage can increase the randomness of an ad's success.
For example, let's say one ad sees an early success. The ad system could then estimate that it is a better ad, and show it in more competitive placements or lower the cost of each impression so it gets shown more often. This gives that ad more opportunities for further success, so it could outperform ads of the same quality.
How It Creates Problems
We want to understand the impact of an ad, not the random events that can't be repeated. This increase in randomness around an ad's performance makes understanding the impact of the ad even harder.
Unfortunately, this increases the randomness in performance for all ad runs. No matter how one runs an ad, there will always be a first $50 spent. What happens in those first few steps often influences how adaptive ad systems treat the ad.
Seeing through the Randomness
Randomized Tests
Oh, randomness. You make the world exciting, and much harder to understand.
Randomness, especially this high-volume form of it, is exactly why we need randomized tests to understand the world. Randomizing an audience across a collection of ads helps reduce the chances that one ad will just get a more interested audience, better placements, or other systematic advantages.
The randomization step also allows us to estimate the uncertainty in our estimates. With a non-randomized run, we know which did better. With a randomized test, we can estimate how likely it is that it ended up this way just because of random events.
Multiple Runs
If we re-run an ad component, we re-run that initial sensitive time (at least partially). So with a few runs, we can separate the impact of ad quality and just good (or bad) luck.
In working to understand the impact of different ad components (visual, headline, targeting) Deciding Data often re-runs an ad a few times. This allows us to separate the impact of each component, and the impact of randomness.
Using Good Luck When You Get It
When testing, we primarily want to understand. But, we also want to improve ad performance. We can use cumulative advantage and lean into it.
When we have an ad that does well. We work to preserve its advantage (reputation on the ad platform) and continue it. Increase the ad budget over time, and in a particular "step-like" way that also avoids biasing our tests. When doing this on an ad platform like Meta's this can cause some re-learning at the different spending levels, but our experience suggests that much of that learned reputation is preserved.
Conclusion
Cumulative advantage can cause an ad to perform notably better or worse based on its initial luck.
This increased randomness in performance is exactly why it is important to use randomized ad experiments. Deciding Data also uses repeated testing to better understand randomness and the impact of a component. We also work to use the good luck you get, my scaling ads that show great performance.